Do AI Models Dream of Accessible Tables?
- 7 November, 2023
- Reading Time: 5 mins
From personalized learning to automated grading, AI’s future in accessibility is promising. It’s already begun contributing to accessibility efforts. So the question in most A11y community chatrooms is just how far can AI go in accessibility. Can AI completely automate accessibility? The short answer: no, not yet. We’ll get into why and how a little later on but first, let’s take a look at how far we’ve come.
Here’s where AI is already making strides in Accessibility
- Automation for Alt Text and Audio Descriptions: AI can help automate some accessibility tasks. It can generate alt text and audio descriptions for images and videos, reducing the need for manual intervention. While it’s not perfect, it offers a promising way to make content more accessible to individuals with visual or hearing impairments.
- Closed Captions Made Easier: AI-driven closed caption generation has been a game-changer. However, it’s not without its quirks; AI may occasionally misinterpret words or context. Nevertheless, it’s a significant step towards making videos accessible to a broader audience.
- Automation for Alt Text and Audio Descriptions: AI can assist in checking and validating color contrast, a crucial aspect of web accessibility. Designers and developers can rely on AI-led color contrast validators to ensure their content complies with accessibility standards.
- Name, Role, and Value Recognition: Machine learning and AI models can recognize the names, roles, and values of web elements. This means improved interaction for users relying on screen readers, as the AI assists in understanding web content.
- Accessible Tables: Complex tables are often challenging for users with disabilities. AI, through machine learning, can assist in generating accessible tables by identifying headers, columns, and rows, making it easier for everyone to access and understand the information presented.
The Role of the Ally In The Loop
Even though we’ve got AI applications that support accessibility testing and remediation, the synergy between AI and human expertise is key. Mutual enhancement is an aspect of AI and human collaboration that could potentially support accessibility. How? When AI models are trained on expert human insights on Accessibility guidelines and checks, it could contribute to more inclusive product and content outcomes. AI can offer insights, but it’s ultimately humans that will refine these insights, providing the necessary depth and context making us instrumental in training AI and ensuring its continued improvement.
Ensuring AI serves accessibility is an ongoing journey, and it depends on the collaboration among developers, accessibility experts, and end-users. Innovation like this could pave the way for more inclusive features such as an accessibility overlay that allows screen reader users to ask questions about an image and get detailed responses. Right now, the challenge lies in training AI models to understand and generate accessible content effectively, and services like a color contrast validator can play a vital role in this process.
Training AI Models for Accessibility
There is a need and importance of training AI on accessible data. The idea of creating universal guidelines for AI dataset ingestion is acknowledged but may take time to develop. Different organizations and individuals have specific data sets and requirements, making it difficult to establish a one-size-fits-all approach.
In practice, it would involve individuals providing AI with datasets that showcase the correct way to present content and the mistakes to avoid. This diverse dataset will be used to train AI models to generate accessible content and should include text, images, and multimedia content. Sources may include websites, documents, and accessibility guidelines.
Once you have your data, it would need to be labeled to distinguish between accessible and inaccessible elements, point out accessibility features, and flag errors. Note that you need to be able to ensure authenticity and secure the rights that you have to reserve that data or the content that you have created. This is crucial to ensure that your output is responsible and ethically sourced.
The annotation process benefits from the expertise of accessibility specialists who can provide detailed and accurate labeling. Also, choosing the right AI model for the task is crucial. Large language models are prime candidates for the job due to their versatility and power.
One way to make products more accessible is by training them with accessible code. Provide examples of both conformant and non-conformant code, allowing AI to learn the difference between what is correct and incorrect in terms of accessibility. End users should work with software engineers, prompt engineers, and others to define their goals and needs. They can also develop criteria checklists based on the desired outcomes and involve different teams to ensure the data is tested and reviewed. To ensure accuracy, trained individuals must review and double-check each other’s work. While the process is ongoing and time-consuming, it is a crucial step toward achieving more accessible AI.
This challenge highlights the complexity of the task, as there is no quick solution to making AI accessible. Moreover, data privacy and ethical usage are also critical concerns so AI should be trained in a manner that respects privacy and adheres to ethical standards.
Collaborative Efforts within the Accessibility Community
Right now, there is a lack of existing collaborative efforts but there are certainly ongoing conversations in the accessibility community. These discussions are crucial, especially among professionals who are passionate about the intersection of AI and accessibility.
Collaborative efforts have the potential to set the foundation for improving accessibility in AI models. While there might not be a dedicated consortium for this purpose, the idea of sharing examples of both non-conformant and conformant code, along with double-checking each other’s work, can help AI learn what is accessible.
These collaborative initiatives can pave the way for the establishment of basic guidelines for AI dataset ingestion. By considering accessibility from the very beginning of AI model development, we can ensure that AI systems generate content that is more inclusive and adheres to accessibility standards.
If you’re interested in specific examples of AI applications in accessibility and how collaborative efforts are shaping the field, watch our MagicA11y Live session on ‘Beyond Aesthetics: Prioritizing Color Contrast for Improved Accessibility.’ This session elaborates on common color contrast issues, testing methods, and the impact of AI in bolstering accessibility.
The future holds exciting possibilities as AI models continue to evolve. By promoting collaboration and developing universal guidelines, we can harness AI’s power to make the digital world more inclusive and accessible for everyone.
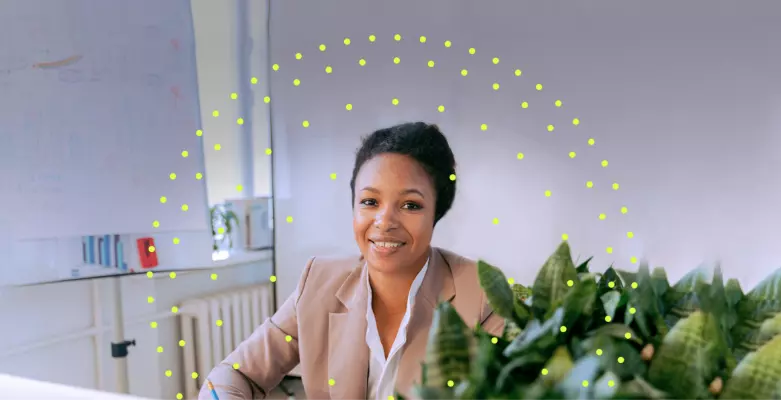